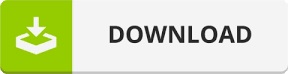
- Admin login sequence diagram install#
- Admin login sequence diagram full#
- Admin login sequence diagram code#
MLflow artifacts can be persisted to local filesĪnd a variety of remote file storage solutions. Refer to Access the MLflow tracking server from outside Databricks, or the quickstart toĮasily get started with hosted MLflow on Databricks Community Edition.Īs mentioned above, MLflow runs can be recorded to local files, to a SQLAlchemy-compatible database, or remotely to a tracking server. HTTP server (specified as which is a server hosting an MLflow tracking server.ĭatabricks workspace (specified as databricks or as databricks://, a Databricks CLI profile. For more details, see SQLAlchemy database uri. Local file path (specified as file:/my/local/dir), where data is just directly stored locally.ĭatabase encoded as MLflow supports the dialects mysql, mssql, sqlite, and postgresql. There are different kinds of remote tracking URIs: To log runs remotely, set the MLFLOW_TRACKING_URI environment variable to a tracking server’s URI or You can then run mlflow ui to see the logged runs. By default, the MLflow Python API logs runs locally to files in an mlruns directory wherever you MLflow runs can be recorded to local files, to a SQLAlchemy-compatible database, or remotely

Once your runs have been recorded, you can query them using the Tracking UI or the MLflow UI let you create and search for experiments. Mlflow.create_experiment(), or using the corresponding REST parameters. You can create an experiment using the mlflow experiments CLI, with You can optionally organize runs into experiments, which group together runs for a Remembers the project URI and source version. If you record runs in an MLflow Project, MLflow ForĮxample, you can record them in a standalone program, on a remote cloud machine, or in an You can record runs using MLflow Python, R, Java, and REST APIs from anywhere you run your code. (for example, a pickled scikit-learn model), and data files (for example, a For example, you can record images (for example, PNGs), models
Admin login sequence diagram full#
MLflow records and lets you visualize the metric’s full history. Each metric can be updated throughout theĬourse of the run (for example, to track how your model’s loss function is converging), and Key-value metrics, where the value is numeric. Key-value input parameters of your choice. Name of the file to launch the run, or the project name and entry point for the run Git commit hash used for the run, if it was run from an MLflow Project.
Admin login sequence diagram code#
Each run records the following information: Code Version MLflow Tracking is organized around the concept of runs, which are executions of some piece ofĭata science code. Using the Tracking Server for proxied artifact access Managing Experiments and Runs with the Tracking Service API Scenario 6: MLflow Tracking Server used exclusively as proxied access host for artifact storage access Scenario 5: MLflow Tracking Server enabled with proxied artifact storage access Scenario 4: MLflow with remote Tracking Server, backend and artifact stores Scenario 3: MLflow on localhost with Tracking Server
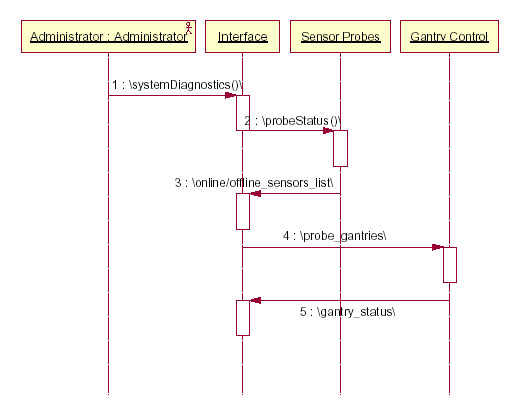
Scenario 2: MLflow on localhost with SQLite Optionally using a Tracking Server instance exclusively for artifact handling.Using the Tracking Server for proxied artifact access.Managing Experiments and Runs with the Tracking Service API.Scenario 6: MLflow Tracking Server used exclusively as proxied access host for artifact storage access.Scenario 5: MLflow Tracking Server enabled with proxied artifact storage access.Scenario 4: MLflow with remote Tracking Server, backend and artifact stores.Scenario 3: MLflow on localhost with Tracking Server.Scenario 2: MLflow on localhost with SQLite.Quickstart: Compare runs, choose a model, and deploy it to a REST API.
Admin login sequence diagram install#
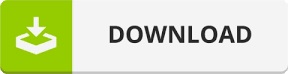